If you’ve ever thought about the life of a graduate student in psychology, you might have pictured someone who is asking research participants probing questions about their hidden thoughts, or perhaps someone who is discreetly observing human subjects completing some tasks while taking quick note of their behavior. In reality, we psychology students spend most of our time learning advanced statistical methods and grappling with quantitative analyses of noisy behavioral data that are difficult to interpret. People not familiar with the norms of the field often find it surprising that mathematics plays a central role in psychological research, as they tend to believe that mathematics is more useful for studying natural sciences that are objective and quantifiable, such as physics, than for studying behavioral sciences that are subjective and not amenable to measurement, such as psychology. In fact, psychology can be just as mathematically rigorous as any of the natural sciences, and the use of mathematical tools is vital to gaining a deeper, precise, and more confident understanding of the human mind as compared to – on the other extreme – armchair psychology that oftentimes leads to wishy-washy interpretations of how humans think. Over the past few decades, major theoretical journals in all subfields of psychology have seen a proliferation of research involving cognitive modeling, which is the formalization of human thinking in terms of mathematical, information-processing language. In this part of the two-part series, I’ll introduce the concept of cognitive modeling and explain the differences between cognitive, conceptual, and statistical models.
To get a quick idea about cognitive models, here’s a brief example of two competing models on how people learn to categorize visual objects. According to the prototype model of categorization, learners abstract and memorize a single summary representation, namely the prototype for each category from all examples already learned in that category. When a new probe item is presented, the similarity of this probe to each category prototype is evaluated, and the category with the most similar prototype is chosen. For example, a prototype-based view on how people categorize a novel-looking pet as a cat or dog would be that the learner first abstracts the mental images of typical dog and cat by morphing all the salient features for both types of the pets, then the learner makes the categorization decision by evaluating the relative similarity of the new pet to the typical dog and cat. In contrast, the exemplar model of categorization specifies that learned examples from all categories were memorized, and the new probe item is classified into the category whose constituent examples are, in sum, most similar to the probe. So, in the example of dog vs. cat categorization, the exemplar-based view would be that the learner makes the categorization decision by comparing the overall similarity of the new pet to the individual examples of both dogs and cats one has seen.

As illustrated by the example above, cognitive models are constructed to provide a mechanistic account of information processing engaged in accomplishing certain cognitive tasks including perceiving, learning, remembering, problem solving and decision making, as well as interaction between these basic cognitive processes.
But what makes cognitive models stand out from alternative approaches to understanding human behavior, namely conceptual and statistical models? One hallmark of cognitive models is that they are described in formal, mathematical or computational, languages. In contrast, conceptual frameworks are merely verbal descriptions of the theoretical understandings. Take the prototype model as an example: a conceptual model would simply state that people form idealized mental images of dog and cat and categorize the novel-looking pet based on how similar it seems to the idealized images. In contrast, the mathematical formulation of the prototype model states that people first specify exact details concerning an ideal mental image of a dog and cat as abstracted from instances of dogs and cats. It also states that they measure and compare the similarities between the ideal dog and cat. One could argue that the conceptual modeling approach can also provide these details by further elaboration, but in my view verbal descriptions can hardly be as precise and elegant as quantitative accounts. For example, the conceptual model could add that the ideal image of a dog should feature the average representations of all the distinctive characteristics shared among dog examples. But such an elaboration still begs the question of how exactly should average representations be defined. In mathematical terms, however, the category abstraction can be concisely defined as the means of all the dog feature values (e.g., tail length, nose depth).
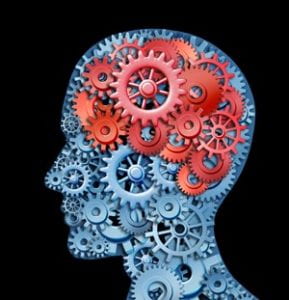
Moreover, the mathematical modeling approach yields very specific predictions about human behavior that can be confidently confirmed or falsified (the same benefit a leger might have over a narrative approximation of expenses). For example, dogs tend to have shorter tails than cats in general, but if a learner happens to see a dog with an unusually long tail, then according to the mathematical definition, the ideal dog image would be certainly changed to have a longer tail. On the other hand, theorists entertaining a conceptual model are free to subjectively decide if an outlier should affect the category ideal and may even disagree among themselves. Some could maintain that the outlier example would distort the summary representation, whereas others could argue that the outlier example should be discounted when constructing a summary representation.
Another hallmark of cognitive models is that they are derived from basic principles of cognition. This is what sets cognitive models apart from statistical models, which generate mathematically optimal solutions to the scientific problems being modeled. Statistical models can be generally applied to data from any field as long as the data meet some fundamental statistical assumptions. However, these assumptions are not founded on any principle of cognition and may even be inconsistent with known facts of cognition. For example, a statistical model of the dog vs. cat categorization would recast the psychological problem into a mathematical one that compares the probabilities with which each identifying feature of a novel pet is sampled from the collections of that feature by dogs versus cats. In particular, the features are always quantified as numeric values (e.g., tail length, nose depth), and for the sake of simplicity and by convention, the feature values for the same category (i.e., pet type) are assumed to be normally distributed. It is noteworthy that the assumption of normal distribution is not necessarily psychological. For one thing, normal distribution embodies the belief that the feature value of all examples of the same pet type should gravitate towards a certain middle value and as the feature value deviates from the middle value the likelihood that the pet with that feature is of the same type drops drastically. In reality, for example, there are quite a number of cat breeds with bobbed tails despite most cats having pretty long tails, so apparently the belief about the middle value doesn’t always reflect our experience with at least certain pet types. On top of that, the normal distribution specifies the notions of the central tendency and variability of feature values in a category as precise numbers, whereas humans tend to think of these concepts on a more gross scale.
Now that you know how cognitive modeling is carried out by psychologists and understand how it stands out from conceptual and statistical modeling approaches to human behaviors, you may wonder why cognitive modeling should be preferred over these other methods. Stay tuned for my next blog that will explain just that.
References:
Busemeyer, J. R., & Diederich, A. (2010). Cognitive modeling. Sage.
Edited by Chloe Holden and Joe Vuletich
Leave a Reply