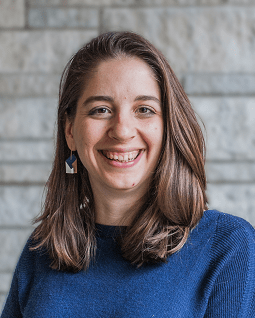
Alice Patania joined the IU Network Science Institute as a research scientist in 2017. She received her PhD in Applied Mathematics at Politecnico di Torino while working at the ISI Foundation in Turin, Italy.
In this Q&A, Patania shares why she decided to take her theoretical mathematics background into the world of complex systems, data science, and higher-order networks and talks about some of the projects she’s working on with Olaf Sporns (IUB Psychological and Brain Sciences) and Liana Apostolova (IUMS Neurology).
Q: What brought you to IUNI?
I did my PhD at ISI in Torino and studied complex systems, and there I started to work on higher-order networks. So in networks, you look at relationships between two actors but in higher-order networks, you are taking into consideration more complex interactions, which happen between three or more actors together. It’s not a concept that is new because, in one way or another, people have been studying them for 50 years, but the research has been very descriptive or case specific and it didn’t generalize well to other areas or applications.
So the idea is to use what has been developed in mathematics over the past 100 years and make it more accessible to researchers in sociology, biology, computer science, and neuroscience—the way it has been done with network science and graph theory—and that’s why I came here. Being at a small institution that specializes in network science would give me the freedom to do higher-order networks because it’s something that’s very new and out there.
Q: Why are you interested in higher-order networks?
There has been very little easy translatable math of higher-order networks—there are a lot of questions that were not easily solvable using math because mathematicians are more interested in finding out how things work and if things work, instead of building a way to make things work, because there might be only one way in a trillion to build it. So I got very intrigued by trying to translate the theoretical insights I had into something that could be useful.
Q: What else are you interested in?
During my theoretical studies, I realized early on I really liked the problem-solving part of mathematics more than writing proofs. I was happy to find out that data science gives me the opportunity to use mathematics to create new ways to study complex problems. Similar to data science, you need a lot of creativity to work in math because you need to think outside the box with something that is not tangible at all. So you’re trying to learn what the function is beyond your data. For me, it’s the most fun.
Q: So what are you working on right now?
A lot of the projects I have are database and data related, so they try to take into consideration an entire network and see the network as a higher-order element of the dataset and then look at the entire collection of networks and study its properties.
One project I’m working on with Olaf (Sporns) is about how brain networks evolve as people get older, or how a healthy person’s brain changes throughout their lifetime. It’s hard to understand the entire development of the changes in connections in a healthy person, while in Alzheimers’ disease you know you’re losing connections. Another project I have is with Liana (Apostolova), where we are trying to find genetic biomarkers in blood for mild cognitive impaired adults associated with Alzheimer’s disease.
Q: What sort of project would you like to work on next?
Since I moved here, I’ve been more and more interested, especially with the work that’s been done at IUNI on the massive Web of Science dataset and other huge datasets, in trying to find ways of building higher-order models that are defined locally, so you can build a higher-order model from what you can see locally in your dataset. It would be a way to describe the entire dataset or network in a way that’s more accessible. You can act on the network locally despite there being no way to look at the entire thing because it’s so huge—so my focus has slightly shifted to these local models for global understanding.
Read more of Alice’s work:
- Topological gene-expression networks recapitulate brain anatomy and function by Patania, A., Selvaggi, P., Veronese, M., DiPasquale, O., Expert, P., & Petri, G.
- The shape of collaborations by Patania, A., Petri, G., & Vaccarino, F.
- Construction of and efficient sampling from the simplicial configuration model by Young, J. G., Petri, G., Vaccarino, F., & Patania, A.
Be sure to follow Alice on Twitter.